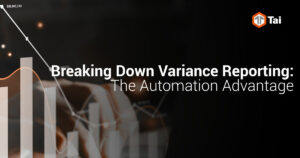
Tai TMS is a comprehensive logistics intelligence system designed to save you time and help scale the growth of your operation. Tai TMS is a multi-modal platform that provides full-scale automation in the shipment lifecycle of both FTL and LTL loads. Through direct integrations with all major carriers and load boards, Tai TMS allows you to go from quote to delivered without manual intervention, empowering your company to dedicate valuable resources to business growth